Challenges in Weather Forecasting: The Butterfly Effect Still Prevails
- Hemadri
- Aug 30, 2022
- 2 min read
The recent increase in extreme weather events such as heavy rainfall, heat waves, cold waves, and tropical cyclones; has made the commoners have a quick look at the TV or the mobile screen for the weather forecast before going out for their daily work. While this is becoming an integral part of the daily routine, the accuracy of weather forecasting hasn’t improved significantly. The science behind weather conditions remains continuously dynamic, chaotic, and non-linear. So, the uncertainty in weather forecasting remains a significant challenge. Before modern-day weather forecasting, the farmers and ship sailors estimated upcoming weather conditions from their past seasonal experiences over time and by observing animals’ peculiar behaviors with respect to weather changes. In 1922, Lewis F. Richardson proposed the idea of Weather Prediction by Numerical Processes, though there was no computational infrastructure to execute those. In this context, during the 1960s, Edward Lorenz coined the phrase "Butterfly Effect", explaining the highly non-linear behavior of the atmospheric processes. In short, he proposed that the weather is so sensitive that even a butterfly's flap could significantly impact weather conditions.
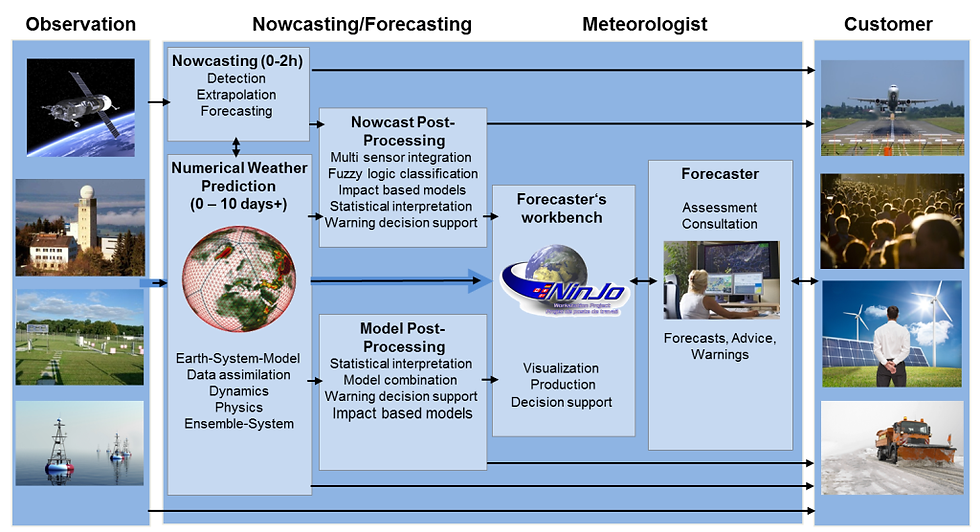
Nevertheless, many significant improvements have been made in weather forecasting in recent years, yet the uncertainty remains. There are some challenges in predicting the actual state of the atmosphere, e.g., the lack of observed in-situ datasets and simulating the out-of-hand growing perturbation in a weather model. To get the forecast right, the present-day numerical weather models need the exact state of the atmosphere in terms of various parameters, which can be achievable via the increase in the number of in-situ observatories across the globe. Right now, we have enough observatories over the land. However, It is challenging to know the exact state of the ocean and atmosphere, and we can't place the instruments all over. And until the present state of the atmosphere is known, one can't predict the future state. Scientists use data assimilation methods to update the system's current state with the latest observation datasets to make it more realistic. The weather models comprise a certain number of dynamical primitive equations. Also, the whole system is imagined as a three-dimensional geoid divided by multiple grid points. Apart from that, many conditions must be taken care of, making the process much more complex and time-consuming. For example, to decrease the forecast error, the weather models must follow a condition following the change in space-time of a fluid parcel to stabilize the weather model, called the CFL criteria. The condition says a modeler must decrease the moving time steps or increase the distance between two grid points. That is one of the reasons we can predict the weather in the short term with better accuracy, from days to weeks in advance, not more than that. Also, we can't predict weather events of short duration because of quick transitioning, for example, the local tornados. Scientists are exploring these aspects for further improvement, and so far, Lorenz's Butterfly Effect is still relevant.
Comments